You stare at the same dashboard for hours. Sales are up this quarter, but was it your new marketing campaign or the seasonal trend? Your client waits for answers, not theories. Definitive proof of what caused what would solve everything.
This challenge, separating true causes from mere correlations, presents itself daily to marketing professionals. Whether you determine which channels drive customer conversion, which creative elements impact engagement, or which audience segments respond to campaigns, mastering causal analysis stands as professionally essential, not just academically interesting.
What Is Causal Analysis?
Causal analysis systematically identifies and understands the true causes behind an effect or outcome. Unlike correlation, which shows that two variables move together, causation establishes that one variable directly influences another.
The refrain “correlation doesn’t imply causation” appears in countless client meetings, but your work demands more than caveats; it requires methods that establish causation.
Understanding Correlation vs. Causation
Correlation is a statistical measure that describes the size and direction of a relationship between two or more variables. When two variables correlate, they tend to move together in a predictable way.
However, correlation only tells us about the relationship between variables, not whether one variable causes another to change. Two variables might be correlated for many different reasons.
Marketing Example:
A strong correlation exists between a company’s social media engagement metrics and their sales figures. As social media engagement increases, sales also increase. This observation alone doesn’t prove that social media engagement causes higher sales.
Causation means that changes in one variable directly bring about changes in another variable. Establishing causation requires more than just observing correlation—it requires understanding the mechanism of influence and ruling out alternative explanations.
Causal relationships have three key characteristics: temporal precedence (cause before effect), covariation (variables change together), and no plausible alternative explanations for the observed relationship.
Marketing Example:
A carefully designed A/B test shows that when identical customer segments receive different email frequencies, those receiving more frequent emails show higher purchase rates. With other factors controlled, we can conclude that increased email frequency causes higher purchase rates for this audience.
Spurious correlations occur when two variables appear to be related but are actually both influenced by a third variable (a confounder) or are related purely by coincidence. These misleading correlations often drive incorrect business decisions.
When you observe a correlation but fail to account for confounding variables, you risk making costly mistakes in strategy and resource allocation.
Marketing Example:
Loyalty program members spend 85% more than non-members, creating a strong correlation between membership and spending. However, this relationship is largely explained by customer interest and past purchasing behavior—customers who already spend more are more likely to join the program. Without accounting for this confounder, you might overestimate the program’s causal impact.
A scenario likely familiar to you: a client’s website traffic and sales increased after implementing a new UX design. The correlation appears clear, but does the redesign actually drive sales? Or does the simultaneously launched email campaign create this effect? Or perhaps an unrelated industry trend explains everything? Proper causal analysis prevents attribution of success to the wrong initiative and misallocation of your client’s future marketing spend.
This distinction between noticing patterns and understanding their causes transforms you from an observer of marketing data to an architect of marketing outcomes. Genuine understanding of causation gives you predictive power over the campaigns you manage.
Why Causal Analysis Matters for Your Agency
The frustration feels familiar: implementing a campaign based on correlational data, only to see disappointing results. This costs your clients money and potentially damages your agency’s reputation.
Consider a retail client that noticed stores with coffee shops had higher sales figures. Based on this correlation, they invested millions installing coffee shops in additional locations. Sales barely budged. Proper causal analysis would have revealed an uncomfortable truth: coffee shops didn’t cause higher sales—both resulted from locations in high-traffic, affluent neighborhoods. The correlation existed, but causal understanding was missing.
This distinction matters enormously in daily agency work:
When clients ask why conversion rates dropped despite increased ad spend, they want to know what to fix, not which metrics moved together.
Deciding between two campaign approaches requires understanding which elements causally drive engagement to make recommendations that deliver consistent results.
Designing new marketing strategies demands knowledge of which touchpoints causally influence purchase decisions to prioritize creative and media resources effectively.
Without causal understanding, high-stakes recommendations proceed in darkness, guided only by patterns that may not represent actionable relationships.
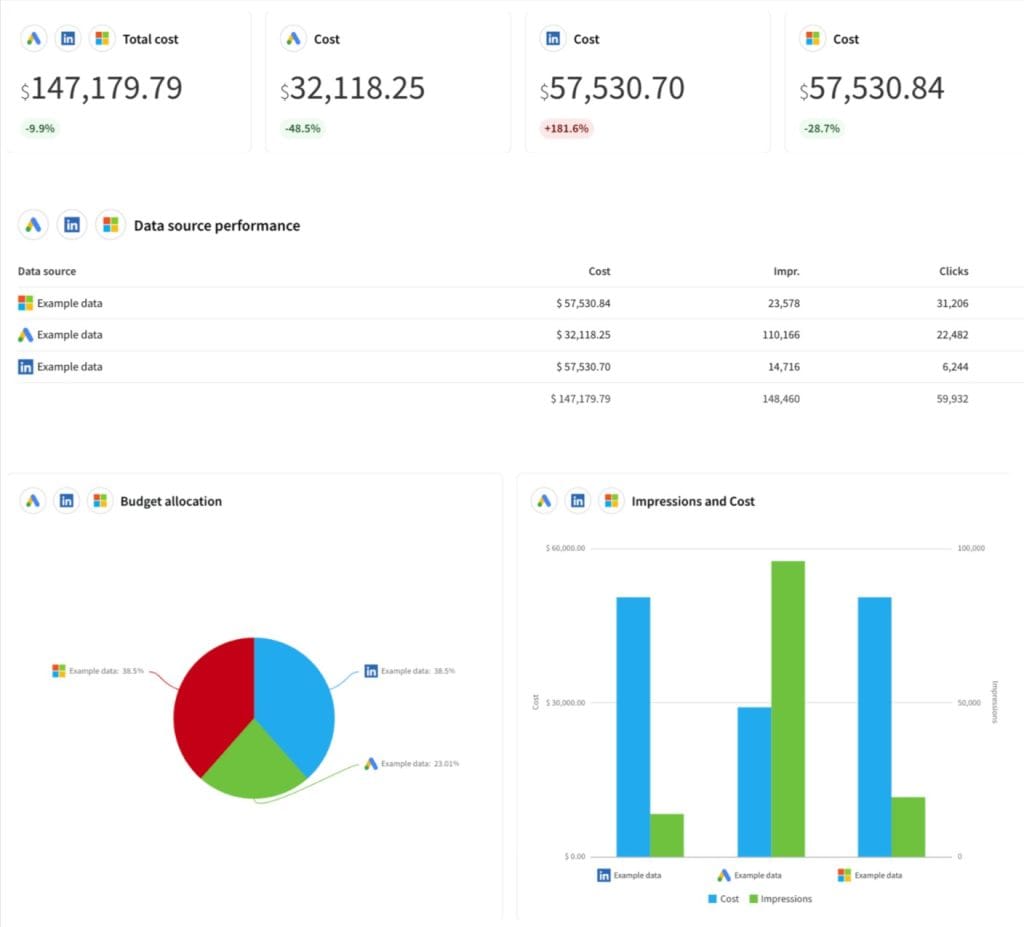
When presenting campaign results to clients, many agencies struggle with fragmented data across multiple platforms. Each ad platform—Google Ads, LinkedIn, Microsoft Advertising—provides its own separate reporting, forcing marketers to manually calculate total spend and compare performance metrics. This fragmentation not only creates unnecessary work but also makes it harder to identify true causal relationships in marketing performance.
Consolidated client reporting tools that combine data sources into unified dashboards provide the foundation needed for proper causal analysis. By seeing the complete picture (total budget, impressions, and conversions across all channels), agencies can better isolate variables and determine which platforms truly drive results versus those that merely correlate with success.
Examples of Causal Analysis in Marketing
Causal analysis transforms marketing decision-making in practice. These concrete examples illustrate the power of moving from correlation to causation in agency contexts.
Casual Analysis Example
From Last-Click to True Impact
A mid-sized agency managed multi-channel campaigns for an e-commerce client with a $2 million annual marketing budget. Traditional attribution models showed social media had the highest ROI, directing increasing budget shares to these channels.
Correlational vs. Causal ROI by Channel
Key Insights:
Social media captured customers who would purchase anyway (selection bias)
Email marketing genuinely caused incremental sales
Budget reallocation based on causal insights increased marketing ROI by 22%
Generated ~$440,000 additional revenue without increasing budget
From Membership Correlation to Causal Value
An agency client implemented a premium loyalty program requiring a $50 annual fee and offering enhanced benefits. Initial comparisons showed members spent 85% more annually than non-members, leading executives to consider heavy investment in member acquisition.
Correlational vs. Causal Spending Lift
Component Effectiveness:
Free Expedited Shipping
High Impact
Exclusive Promotions
Medium Impact
Early Access to Products
Low Impact
Result: Similar lift at 30% lower cost per member
From Creative Testing to Causal Understanding
An agency managing a large CPG brand’s digital campaign needed to optimize creative elements across audience segments. Traditional A/B testing identified winning variations but couldn’t explain performance differences or leverage insights for future campaigns.
Creative Element Effectiveness by Segment
Key Findings:
Emotional messaging had 3x greater impact on new vs. loyal customers
Product imagery strongly affected price-sensitive segments only
Simple messages for occasional buyers, detailed for frequent purchasers
Resulting campaigns improved conversion rates by 24%
The Foundation: Causal Frameworks You Can Apply
Conceptual frameworks transform how you think about causality in marketing work. Two approaches revolutionized causal analysis in recent years, providing powerful mental models for immediate application.
The Ladder of Causation
Judea Pearl’s “Ladder of Causation” framework helps identify your level of causal reasoning:
Judea Pearl’s Ladder of Causation
Observing correlations and patterns in data without making causal claims.
Example: “Does premium ad placement correlate with higher click-through rates?”
Methods: Statistical analysis, correlations, regression
Understanding what happens when you take specific actions or interventions.
Example: “Will moving this client to premium ad placements increase their CTR?”
Methods: A/B testing, quasi-experiments
Reasoning about what would have happened under different circumstances that didn’t actually occur.
Example: “Would campaign X have performed better with different creative?”
Methods: Structural causal models, advanced counterfactual methods
Level 1 likely feels comfortable—most marketing analytics operates there. But client questions (“What happens if we change our media mix?”) exist at Level 2. Deep strategic insights (“What would have happened had we launched on TikTok instead of Instagram?”) require Level 3 thinking.
This distinction helps match analytical approaches to causal questions clients want answered. Client inquiries about upticks in customer engagement demand intervention-level causal understanding, not correlation coefficients.
The Potential Outcomes Framework
Statistician Donald Rubin’s framework conceptualizes causal effects as the difference between potential outcomes under different treatments. This acknowledges a fundamental marketing challenge: you never observe both outcomes for the same campaign.
The Potential Outcomes Framework
The Fundamental Problem of Causal Inference in Marketing
Customer Before Campaign
Receives Campaign
Potential Outcome Y(1)
Observable outcome after receiving campaign
Does Not Receive Campaign
Potential Outcome Y(0)
Counterfactual outcome without campaign
Causal Effect Formula
The true causal effect is the difference between the two potential outcomes
The Fundamental Challenge
We can only observe one potential outcome for each customer. The other outcome remains counterfactual – what would have happened in an alternate reality.
Solutions in Marketing
A/B Testing with Random Assignment
Matched Control Groups
Natural Experiments
Launching a campaign to a customer segment allows observation of what happens when they receive it, but prevents simultaneous observation of what would have happened without it. This “fundamental problem of causal inference” explains why establishing causation exceeds the difficulty of finding correlation—and necessitates specialized methods to overcome this challenge.
These frameworks provide practical clarity when designing analyses that answer causal questions clients prioritize.
Practical Causal Analysis Frameworks for Marketing
While conceptual frameworks help us think about causality, marketing professionals need specific methodologies they can apply to client problems. These six practical frameworks provide structured approaches to identifying and validating causal relationships in marketing contexts, each suited to different situations and data availability.
Six Causal Analysis Frameworks for Marketing
From simple root cause analysis to advanced statistical methods
5 Whys
Simple iterative technique to identify root causes by repeatedly asking “why” until reaching the underlying issue
Fishbone Diagram
Organizes potential causes into categories to provide a structured approach to identifying multiple contributing factors
Counterfactual Analysis
Examines “what would have happened if X had not occurred?” by creating alternative scenarios to compare with observed outcomes
Causal Loop Diagrams
Visualizes how variables in a system influence each other through feedback loops, revealing reinforcing and balancing effects
Impact Mapping
Strategic planning technique that connects business goals, actors, impacts, and deliverables to identify causal paths to desired outcomes
Directed Acyclic Graphs
Causal diagrams that use nodes and directed edges to represent variables and their causal relationships with mathematical rigor
Framework Comparison
Compare key characteristics to select the right tool for your analysis
Framework | Best For | Data Requirements | Team Involvement | Time Investment | Statistical Rigor |
---|---|---|---|---|---|
5 Whys | Quick root cause analysis of specific issues |
Low |
High |
Low |
Low |
Fishbone Diagram | Comprehensive brainstorming of multiple causes |
Low |
High |
Medium |
Low |
Counterfactual Analysis | Quantifying the impact of specific factors |
High |
Medium |
Medium-High |
High |
Causal Loop Diagrams | Understanding systemic effects and feedback loops |
Medium |
Medium |
Medium-High |
Medium |
Impact Mapping | Planning interventions based on causal assumptions |
Low |
High |
Medium |
Low |
Directed Acyclic Graphs | Rigorous analysis of causal relationships |
High |
Low |
High |
Very High |
Framework Selection Tool
Answer these questions to find the most appropriate framework
What is your primary goal?
How much data do you have available?
What time constraints are you working with?
Who will be involved in the analysis?
Recommended Framework
5 Whys
Based on your answers, the 5 Whys framework is most appropriate for your situation. This simple but effective technique will help you quickly identify root causes with minimal data requirements.
Why this recommendation?
- You need to diagnose a specific problem
- You have minimal data available
- You need a solution quickly
- It works well for individual analysis
Interactive Case Study: Mountain View Digital Agency
See how each framework helps solve a real marketing challenge
The Scenario
Mountain View Digital Agency is working with CleanCare, a D2C home cleaning products brand facing declining conversion rates despite increased ad spend. The agency needs to identify the true causes of this decline.
-23%
Conversion Rate
+35%
Ad Spend
+75%
CAC
±0%
AOV
+42%
Traffic
82%
Cart Abandonment
Why are conversion rates declining despite more traffic?
New visitors are abandoning carts at a higher rate than before.
Why are visitors abandoning carts more frequently?
Customers are experiencing unexpected shipping costs at checkout.
Why are shipping costs surprising customers?
CleanCare recently changed their free shipping threshold from $35 to $75.
Why did CleanCare change their free shipping threshold?
Increased shipping and fulfillment costs reduced margins, prompting the change.
Why wasn’t this change communicated effectively to customers?
The marketing team wasn’t involved in the operational decision, so promotional materials and ads still implied more favorable shipping terms.
Root Cause:
Cross-departmental communication breakdown between operations and marketing led to misaligned customer expectations and actual shipping policy.
Recommended Solution:
Update all marketing materials to clearly communicate shipping costs, test a tiered shipping approach (e.g., $5 shipping for orders $35-$74, free over $75), and implement cross-functional decision processes for customer-facing changes.
Declining conversion rates despite increased ad spend
People
- New marketing team members lack experience
- Recent loss of senior CRO specialist
- Team workload preventing thorough analysis
Process
- New product launch process bypassed usual testing
- Checkout process added additional steps
- Shipping policy changes not reviewed by marketing
Technology
- Website redesign introduced new friction points
- Mobile site performance issues
- Tracking pixel errors causing data gaps
Communication
- Inconsistent messaging across channels
- Shipping costs not clearly communicated
- Value proposition less prominent in new designs
Competition
- Major competitor launched free shipping on all orders
- New market entrant with aggressive promotions
- Competitor improved product formulations
Key Insights:
The fishbone analysis revealed three primary areas to investigate further:
- The shipping policy change coincided with the conversion decline
- Recent website changes created new friction in the checkout process
- Competitor’s free shipping offer changed market expectations
“What would conversion rates have been if the shipping policy hadn’t changed?”
Treatment Group
All website visitors after shipping policy change
3.1%
Conversion Rate
Control Group
Customers who received free shipping promotions
4.2%
Conversion Rate
Estimated Causal Effect
-1.1percentage points
This accounts for approximately 48% of the total conversion decline
Conclusion:
The counterfactual analysis confirms that the shipping policy change was the primary driver of declining conversions, with website friction as a secondary factor. The data suggests restoring the previous shipping threshold would recover approximately half of the lost conversion rate.
Reinforcing Loop (R1): The Value Perception Cycle
Same direction change
Opposite direction change
Insight from CLD:
The diagram reveals that the shipping cost change triggered multiple reinforcing loops that amplify its negative effects. Simply increasing ad spend temporarily addresses symptoms but doesn’t fix the underlying value perception issue, while simultaneously strengthening the negative reinforcing loops.
Recommended Intervention:
Break the reinforcing loops by implementing tiered shipping with a lower initial threshold, enhancing product value perception, and creating loyalty programs that offset shipping costs for repeat customers.
Increase conversion rate by 30% within 90 days
Goal (Why)
First-time visitors
Price-sensitive customers
Impacts (How)
Reduce perceived checkout friction
Improve price-value perception
Deliverables (What)
Simplified checkout process
Transparent shipping calculator early in process
Tiered shipping costs instead of all-or-nothing threshold
Bundle options that reach free shipping threshold
90-Day Implementation Plan:
- Implement tiered shipping costs (immediate)
- Add transparent shipping calculator to product pages (2 weeks)
- Create product bundles that reach free shipping threshold (4 weeks)
- Launch simplified checkout process (8 weeks)
- Develop loyalty program with shipping benefits (12 weeks)
Economic Conditions
Price Sensitivity
Competitor Actions
Traffic Quality
Shipping Cost Policy
Website Redesign
Cart Abandonment
Statistical Testing Results:
Shipping cost policy impact
Website redesign issues
External factors & measurement error
Essential Steps to Perform a Causal Analysis That Delivers Results
The practical steps of conducting a causal analysis that withstands scrutiny and delivers actionable insights for marketing campaigns follow a clear path. These approaches work with your current team, regardless of technical background.
The 7-Step Causal Analysis Process
Your causal question must specify:
- The exact intervention (e.g., “increasing email frequency from weekly to daily”)
- The specific outcome (e.g., “conversion rate”)
- The population of interest (e.g., “enterprise customers”)
This precision determines everything that follows in your analysis.
A DAG forces articulation of assumptions about how variables influence each other. This visualization shows:
- Direct causes and effects
- Confounding variables (factors influencing both cause and effect)
- Mediating variables (pathways through which causes produce effects)
This critical step identifies potential sources of bias in your analysis.
Options range from experimental to quasi-experimental approaches:
- Randomized Experiments: The gold standard when feasible
- Difference-in-Differences: For before-after comparisons with control groups
- Regression Discontinuity: For threshold-based treatments
- Propensity Score Matching: For comparing similar treated and untreated units
Your choice depends on data availability, ethical considerations, and practical constraints.
Prioritize:
- Measuring all confounders identified in your DAG
- Establishing temporal sequence (causes must precede effects)
- Adequate sample size to detect meaningful effects
- Consistent measurement procedures to minimize error
No statistical approach can overcome fundamental data limitations.
Implementation principles include:
- Explicitly controlling for confounding variables
- Conducting sensitivity analyses to assess robustness
- Examining effect heterogeneity across subgroups
- Assessing both statistical and practical significance
Document analytical decisions transparently for credibility.
Causal interpretation requires:
- Acknowledging limitations of your identification strategy
- Being precise about what results do and don’t tell you
- Considering context and generalizability
- Using language that accurately reflects evidence strength
Most importantly, translate findings into clear decision implications for stakeholders.
No single analysis provides definitive causal evidence. Consider:
- Implementing multiple analytical approaches
- Testing additional implications of your causal theory
- Seeking natural experiments that provide alternative identification
- Comparing findings to established theoretical predictions
Convergent results from different methods with different assumptions strengthen causal claims.
Step 1: Define Your Causal Question with Precision
A precisely defined question must start your causal analysis—one that explicitly addresses causation, not just association. The difference appears subtle but proves crucial.
Compare these questions: “Is there a relationship between our email frequency and conversion rate?”
Versus: “Does increasing email frequency from weekly to daily cause an increase in conversion rate for our client’s enterprise customers?”
The second question specifies:
- The exact intervention (increasing email frequency from weekly to daily)
- The specific outcome (conversion rate)
- The population of interest (enterprise customers)
This precision determines everything that follows. Countless agency analyses fail because vague causal questions lead to methods that miss what clients need to know.
Formulating your causal question requires asking:
- What specific recommendation will this analysis inform?
- What intervention does the client consider?
- What outcome does the client want to influence?
- Which audience segment needs this information?
Precise causal questions focus your analysis and ensure results directly inform client recommendations.
Step 2: Map the Causal Relationships in Your Marketing System
A critical step many marketers skip comes next: creating a visual representation of potential causal relationships. Directed acyclic graphs (DAGs) force articulation of assumptions about how variables in your marketing system influence each other.
Analysis of whether a client’s loyalty program causes increased customer lifetime value might produce this simplified DAG:
Customer Interest → Program Enrollment → Customer Lifetime Value
↓ ↑ ↑
└───────→ Previous Purchase History ────────┘
↑
│
Brand Perception
This visualization shows:
- Customer interest affects both program enrollment and CLV directly
- Previous purchase history influences program enrollment and CLV
- Brand perception influences purchase history
- Program enrollment may affect CLV (the relationship under investigation)
Creating this diagram helps you think carefully about confounding variables—factors that influence both cause and effect, potentially creating false connections. If you don’t account for factors like customer interest and purchase history, you might assign effects to the wrong causes and make poor recommendations to clients.
Simple diagramming tools or hand-drawn diagrams are enough; the thinking process itself is valuable. Consultation with client marketing teams and review of industry research ensures your DAG captures key relationships in their marketing system.
Step 3: Choose Your Analysis Strategy Based on Your Constraints
Your DAG identifies potential confounding variables and guides appropriate analysis strategy selection. Options range from experimental approaches (gold standard) to quasi-experimental methods suited for observational data (marketing reality).
Randomized Experiments: The Gold Standard When Feasible
Random assignment of marketing interventions—whether email messages, display ads, or special offers—creates comparable groups differing only in intervention receipt. This eliminates confounding and provides clear causal evidence.
Digital marketing makes A/B testing increasingly accessible. Causal questions about website design, email campaigns, or ad creative often permit experimental approaches. Many clients don’t realize how easily these tests integrate with existing marketing technology.
Quasi-Experimental Methods: Your Practical Toolkit
When randomization proves impossible (common for brand campaigns, channel strategies, or loyalty programs), quasi-experimental methods approximate experimental conditions:
- Difference-in-Differences: Before-and-after data for exposed and unexposed markets isolates causal effects through comparison of changes over time. Campaign launches, market entries, or channel expansions affecting some regions but not others benefit from this approach.
- Regression Discontinuity: Treatment assignment based on thresholds (spending levels or engagement scores) allows comparison of units just above and below thresholds to reveal causal effects. Loyalty tier benefits, threshold-triggered offers, and qualification-based programs work well with this method.
- Instrumental Variables: Variables affecting marketing exposure but not outcomes directly isolate causal effects. Marketing contexts make finding valid instruments challenging.
- Propensity Score Matching: Matching exposed customers with similar unexposed customers based on exposure probability works especially well with rich customer data when experiments prove impossible.
Context determines choice: available client data, timeline and budget constraints, and causal question nature all matter. Simple methods addressing key confounders often prove most effective for agency work.
Causal Analysis Methods Comparison
Randomized Controlled Trials (A/B Testing)
The gold standard for causal inference. Random assignment of treatment ensures that confounding variables are balanced between groups, allowing for direct measurement of causal effects.
Best For:
Digital marketing interventions, email campaigns, landing page optimization, ad creative testing, and other contexts where random assignment is ethical and practical.
Difference-in-Differences
Compares the changes in outcomes over time between a group that received treatment and a group that did not. Controls for time-invariant confounders and common time trends.
Best For:
Evaluating marketing campaigns launched in some markets but not others, assessing channel expansions, and analyzing policy changes when before-and-after data is available.
Regression Discontinuity
Uses a threshold or cutoff point in an assignment variable to compare units just above and below this threshold. Units near the cutoff are nearly identical except for treatment status.
Best For:
Loyalty tier programs, threshold-based offers or discounts, qualification-based marketing programs, and any intervention assigned based on a clear cutoff value.
Propensity Score Matching
Matches treated units with similar untreated units based on their likelihood of receiving treatment given observed characteristics. Creates comparable groups for causal comparison.
Best For:
Evaluating self-selected programs like loyalty memberships, opt-in email campaigns, or any marketing context with rich customer data but without random assignment.
Step 4: Collect Data That Actually Supports Causal Inference
Data collection strategy determines causal analysis quality. Sophisticated methods cannot overcome fundamental data limitations.
Marketing causal analysis data collection priorities include:
- Measuring confounders: DAG-identified confounding variables must appear in your data. Omitted variable bias from unmeasured confounders like brand preference, competitive exposure, or previous purchase intent causes most marketing analysis failures.
- Temporal sequence: Your data must show that marketing exposure happened before the outcomes. Data collected over time at multiple points helps track how customer behavior changes after they see a campaign.
- Sample size planning: Power analyses ensure samples detect effect sizes that matter. Studies with too few participants waste client money on analyses that can’t answer their questions.
- Consistent measurement: Validated metrics and consistent measurement procedures minimize measurement error that attenuates causal estimates.
Many agencies invest heavily in sophisticated analytical methods while neglecting data quality.No statistical method can fix basic data problems—your conclusions about causes can only be as good as your data collection approach.
Step 5: Implement Your Analysis with Appropriate Rigor
Question definition, relationship mapping, strategy selection, and data collection prepare you for analysis implementation. Technical details vary by method, but several principles apply broadly to marketing analytics:
First, control explicitly for DAG-identified confounding variables. This may involve regression adjustment, matching, weighting, or stratification, depending on analytical approach.
Second, conduct sensitivity analyses to assess finding robustness against assumption violations. Ask how strong unmeasured confounding must be to explain away effects and how much measurement error would change conclusions.
Third, assess practical significance beyond statistical significance. Statistically significant conversion rate lifts might prove too small to justify campaign costs. Practically significant effects might warrant action despite somewhat ambiguous statistical evidence.
Fourth, examine causal effect heterogeneity across audience segments or contexts. Average effects might mask important variations that inform more targeted campaigns.
Fifth, document analytical decisions transparently, including alternatives considered and sensitivity checks performed. This builds finding credibility when presenting to clients and provides interpretation context.
Step 6: Interpret Results Through a Causal Lens
Result interpretation requires precision and caution. Agencies commonly overextend causal claims beyond what analyses actually support.
Clarity about what results do and don’t tell you matters:
- When estimating average effects, you need to recognize that individual results can vary greatly across different customer groups.
- Analyses relying on specific assumptions must acknowledge assumption plausibility in client contexts.
- Causal identification from particular subpopulations (customers near loyalty thresholds) demands caution when generalizing beyond this subpopulation.
Your words should match how strong your evidence is. Words like ‘suggests,’ ‘provides evidence for,’ or ‘demonstrates’ show different levels of certainty that should match how solid your methods are.
Most importantly, translate causal findings into clear campaign implications. Clients need to understand what findings mean for marketing decisions, not statistical details. “Increasing email frequency causes a 2.3 percentage point increase in conversion rates for enterprise customers” translates to: “Increasing email frequency for enterprise customers will likely improve conversion rates, but pilot this change with a subset before full implementation.”
Causal Claims Strength Scale
Choose language that accurately reflects the strength of your causal evidence
When to use: Observational studies with limited control for confounders, or small sample exploratory analyses.
When to use: Well-designed quasi-experimental methods or observational studies with robust controls for confounding.
When to use: Well-executed randomized controlled trials or convergent evidence from multiple robust methods.
Step 7: Strengthen Your Case Through Triangulation
No single analysis provides definitive causal evidence. Multiple lines of evidence using different methods with different assumptions and potential biases support compelling causal claims.
Multiple analytical approaches showing similar conclusions strengthen findings. Testing additional causal theory implications (if campaign A causes awareness lift, consideration effects should follow) adds support. Natural experiments or market changes providing alternative causal identification strategies further reinforce conclusions.
Loyalty program customer retention impact studies might use propensity score matching to compare members and non-members, implement regression discontinuity design around qualification thresholds, examine outcomes after policy changes expanding program eligibility, and compare findings to industry benchmarks for similar programs.
Convergent results from multiple methods with different assumptions translate to stronger causal claims and more successful marketing recommendations.
Avoiding the Common Pitfalls That Derail Marketing Analyses
Causal analyses challenge even seasoned marketers. Awareness of common pitfalls prevents them in client work.
Confusing Correlation with Causation: The Fundamental Error
The conceptual distinction between correlation and causation proves easy to overlook in practice. Correlation arises from multiple causal structures:
- Direct causation (Marketing causes Purchase)
- Reverse causation (Purchase intent causes marketing exposure)
- Common causes (Brand affinity causes both marketing exposure and purchase)
- Coincidence (particularly with small samples or multiple testing scenarios)
Agency contexts demand vigilance regarding alternative explanations for observed associations. DAGs identify and test competing causal hypotheses that might explain client data patterns.
Omitted Variable Bias: The Silent Threat to Marketing Claims
When you leave out important factors that influence both variables, you create what’s called ‘omitted variable bias’—a type of error that can lead to wrong conclusions and marketing recommendations that don’t work.
Social media campaign effect analyses often neglect brand preference. People already preferring a brand more likely engage with its social content and purchase its products, creating spurious association between social engagement and purchase even when social content lacks causal effect.
To protect against omitted variable bias, you need to carefully think about all possible factors that might influence your results and collect data on them. When you think there might be unmeasured factors (which is common in marketing), you should run tests to see how strong these hidden factors would need to be to make your conclusions wrong.
Selection Bias: When Your Marketing Audience Misleads
If your analysis sample is too different from your target audience, you can’t apply your conclusions to that audience. Selection bias particularly plagues marketing when selection processes relate to both exposure and outcome.
This problem is clear in loyalty program studies that only look at customers who stayed active for six months after joining. If customers who didn’t benefit from the program quit earlier, studying only the customers who stayed will make the program look more effective than it really is.
Selection bias mitigation requires intentional sampling strategies, documentation of selective attrition or participation, and analytical approaches accounting for sample selection when making client recommendations.
Post-Treatment Bias: Controlling for the Wrong Marketing Variables
Not all control variables improve causal estimation; controlling for variables affected by marketing exposure distorts causal estimates. DAGs identify “downstream” treatment variables that should not receive control.
Studies of brand campaign effects on purchase intent shouldn’t control for brand awareness (a potential mediator through which campaigns affect purchase intent). Such control inappropriately “controls away” campaign effects under investigation.
The key principle: control for confounders (common causes of marketing exposure and outcomes) but avoid controlling for mediators (variables through which marketing affects outcomes) or colliders (common effects of marketing and outcomes).
Overfitting Causal Models: When Complexity Obscures Marketing Causality
Complex models with many parameters risk capturing noise rather than true causal relationships. Predictive modeling often benefits from complexity, but causal inference typically requires parsimonious approaches maintaining clearer causal interpretation.
Causal analysis aims not at perfect customer behavior prediction but at understanding specific marketing action and outcome causal relationships. Simpler models with clearer causal interpretations often outperform complex models that fit data slightly better but obscure causal mechanisms.
Advanced Techniques That Give Your Agency an Edge
Once you understand the basics of causal inference, you can start using more advanced methods that give deeper insights or answer complex marketing questions. Better software and more available resources have made these advanced approaches easier to use.
Machine Learning for Causal Inference: Combining Flexibility with Rigor
Recent advances combine machine learning flexibility with causal inference interpretability:
- Causal Forests extend random forests to estimate heterogeneous treatment effects—effect variation across customer segments. This identifies audiences benefiting most from specific campaigns, enabling more targeted marketing.
- Double/Debiased Machine Learning uses machine learning for nuisance parameters while maintaining valid causal parameter inference. This controls for numerous potential confounders without overfitting.
- Meta-Learners estimate treatment effects from flexible prediction models, bridging predictive and causal modeling. X-Learners and R-Learners appear in increasingly accessible packages.
These approaches excel with numerous potential confounders or complex, non-linear variable relationships—situations common in modern marketing analytics with rich customer data.
Mediation Analysis: Understanding the ‘How’ Behind Marketing Causation
Campaign effectiveness investigations sometimes require understanding mechanisms, not just effects. Mediation analysis decomposes total effects into direct and indirect components, identifying pathways through which marketing produces effects.
New content strategy conversion rate improvements might work through:
- Stronger brand perceptions
- Clearer product benefits reducing purchase uncertainty
- Emotional connections driving preference
Understanding these mechanisms refines campaigns, strengthening important pathways while eliminating costly components that don’t contribute to causal effects.
Modern mediation analysis approaches provide more robust causal pathway identification frameworks than traditional approaches, deepening insight into marketing effectiveness mechanisms.
Synthetic Controls: Solutions for Marketing Case Study Challenges
Case studies lacking traditional control groups, such as major rebrand or market entry analyses, benefit from synthetic control methods:
- Synthetic comparisons (what would have happened without marketing intervention) created from weighted combinations of untreated markets or time periods
- Pre-intervention trend matching establishes credible counterfactuals
- Visually compelling, intuitive results communicate clearly to clients
This approach evaluates unique marketing interventions where traditional experimental or quasi-experimental designs prove infeasible, adding powerful tools to your causal toolkit.
Time Series Causal Analysis: Tackling Temporal Marketing Complexity
Time series data—common in campaign tracking, sales analysis, and media measurement—requires specialized causal methods addressing temporal dependence challenges:
- Granger Causality tests whether past marketing metric values predict future performance metric values, accounting for target variable history
- Structural Vector Autoregressions model contemporaneous causal relationships in marketing systems
- Interrupted Time Series analyzes changes following campaign launches or major marketing events
- Bayesian Structural Time Series creates synthetic controls for time series causal inference
These methods disentangle complex temporal relationships and identify causal effects in dynamic marketing systems where traditional cross-sectional approaches fail.
Conclusion
Causal analysis represents a powerful approach transforming agency recommendations and client value delivery, not just an academic exercise.
The difference between correlation and causation is crucial—it’s the difference between seeing patterns and understanding why things happen. This understanding enables effective campaign design and marketing action consequence prediction with greater confidence.
Rigorous causal analysis requires careful thought and specialized techniques, but fundamental principles remain accessible regardless of technical background. Key insights for agency analytical practice include:
- Start with precise causal questions specifying marketing interventions and outcomes of interest
- Visualize marketing system causal relationships using directed acyclic graphs (DAGs)
- Select analysis strategies addressing client context confounding challenges
- Collect data designed intentionally to support causal inference
- Interpret results cautiously and translate them into clear recommendations
- Strengthen causal claims through multiple method triangulation
Causal reasoning skill development changes questions about marketing data. Moving from “What patterns exist?” to “What happens if we implement this campaign?” shifts perspective from association to intervention to counterfactual reasoning. This elevates strategic contributions and improves client outcomes.
Causal analysis combines rigorous methodology with marketing expertise and critical thinking. Strong marketing analyses address specific causal questions most relevant to client business objectives, not technical sophistication.
Marketing data-based recommendations demand consideration of causal structures underlying observations. Questioning pattern existence, reasons, and intervention responses prevents costly mistakes, identifies effective campaign strategies, and drives superior client results.
Move from correlation to causation in your marketing analytics.
Start Your Free Trial Today