You work in marketing, so you already know the pressure is on to get results. Clients want more than just creative ideas; they want to see a real return on their investment. In a world overflowing with data, relying on gut feelings and creative sparks won’t get you very far. To truly succeed, understand data—and that means knowing the difference between parameters and statistics.
The Marketing Challenge
A new client walks in the door. It’s a cool new footwear company that wants to reach young professionals. They have awesome products, but their past marketing hasn’t worked. You need a strategy that wins, and you need it now.
First, understand your target audience—what they like, how they act, what motivates them, and their problems. How can you get this valuable information without spending a fortune or wasting time on a wild goose chase for data?
Trying to get a survey to every single young professional who might like your client’s shoes is like trying to herd cats. It takes forever, costs a ton, and honestly, it’s impossible. Your clients wouldn’t be happy with that approach, and your budget would definitely take a hit.
Statistics become your best friend. They offer a practical way to gather important information without emptying your wallet or overworking your team. When you understand how statistics connect to the bigger idea of parameters, you can use data to make smart marketing choices that actually work.
Now that you understand the challenge, let’s look at what makes up marketing data.
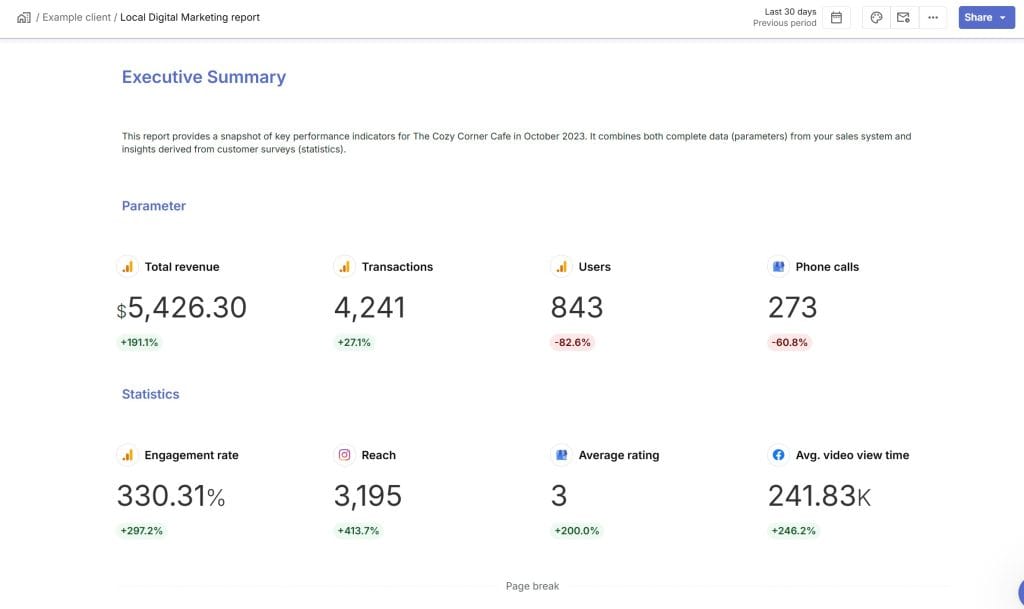
What Are Parameters?
Think of a parameter as the ultimate truth in marketing data. It’s the definitive answer to your marketing questions and the key to really understanding your target audience. A parameter represents a characteristic of an entire population—the exact value you would find if you could measure every single person in the group you’re interested in.
Here are some important parameters for marketers:
- Average customer lifetime value: This is the gold mine. Understand how much money a customer will bring in over their lifetime to justify your marketing spend to clients and demonstrate long-term value. Confidently tell your client, “Our data shows that each new customer we get from this campaign will probably spend about $500 with us over the next three years.” That’s the power of understanding customer lifetime value.
- Overall brand awareness: In a market with lots of competition, brand awareness is key. Know how many people recognize your client’s brand to see how well your campaigns are doing and where you can improve. Want to get your client’s brand noticed by more young professionals? Track a parameter like “the percentage of young professionals who can name your client’s brand when given a list of competitors.” This becomes a critical number for your strategy.
- Conversion rate across all channels: This is what it all comes down to. Drive sales, generate leads, or increase website traffic—conversion rates show how successful you are. If you’re running a campaign across different channels, find out the actual conversion rate for each one—email, social media, paid ads, and so on. This helps you spend your money wisely and focus on the channels that give you the best return.
Finding these exact parameters is often like finding a needle in a haystack. You’re dealing with a huge group of people, and it’s just not possible to get data from every single one of them.
What is Statistics in Marketing?
Getting complete data sounds perfect, but there’s a more practical approach.
While parameters might seem ideal, statistics are the practical solution—and that’s a good thing! Statistics are your secret weapon, the tool that helps you tackle the challenges of marketing. They help you make smart choices based on real data, not just your instincts.
Analyze data from a small but representative group of your target audience to calculate statistics. This gives you a surprisingly accurate estimate of those hard-to-find parameters.
Collect Sample Data (e.g., Survey 500 customers)
Analyze & Find Patterns (e.g., 80% prefer mobile shopping)
Test Findings (e.g., Optimize mobile experience)
Scale Success (e.g., Roll out to all customers)
For example, determine how many likes, comments, and shares the average Instagram post about healthy food gets. Instead of looking at every single post about healthy food on Instagram (which would be impossible!), select a representative sample of, say, 1,000 posts from different accounts and calculate their average engagement. This statistic from your sample would give you a good idea of the engagement for all healthy food posts on Instagram.
Here’s how statistics can help you solve some common marketing problems:
Marketing Goal | Sample Statistic | Insights Gained | How to Collect |
---|---|---|---|
Increase how many people open your emails | Look at the open rate of a small test email campaign | This helps you guess how many people will open your emails when you send them out to everyone | Test different subject lines on a small part of your email list |
Get more people to click on your ads | Check the click-through rate of a test Facebook ad campaign | This helps you predict how many people will click your ads if you spend more money on them | Run a small test campaign with different ad designs and ways of targeting people |
Get more people to take action on your website | See how many people take action on a new version of your landing page | This helps you guess how much your website actions will increase if you change your whole website | Test different versions of a landing page to see which one works best |
Use these sample statistics to make decisions based on data to improve your marketing and get the most out of your money—no magic needed!
Here’s a practical guide to when and how to use each type of data in your marketing:
Marketing Data Guide: When to Use What
Statistics (Sample Data)
Campaign Testing
• A/B test email subjects (1,000 subscribers)
• Social media ad variations (5% of budget)
• Landing page variants (split traffic)
Market Research
• Customer satisfaction surveys
• Product feedback interviews
• Competitor analysis
Best for: Quick insights & testing
Watch out for: Sampling bias
Parameters (Complete Data)
Financial Metrics
• Total revenue
• Customer lifetime value
• Campaign ROI
Platform Analytics
• Email list size
• Website conversion rates
• Social media follower count
Best for: Critical business decisions
Watch out for: Time & cost constraints
What’s the Difference Between a Parameter and a Statistic?
We’ve talked about what parameters and statistics are, but let’s make sure we’re clear on the differences between these two important ideas:
Parameter | Statistic |
---|---|
Describes something about an entire population | Describes something about a sample |
Uses Greek letters (like μ for the population mean) | Uses regular letters (like x̄ for the sample mean) |
Has one fixed value (there’s only one true value for the population) | Can have different values depending on the sample |
Usually hard to measure directly because you need data from everyone | Calculate it from the data you collect from your sample |
The real value you’re trying to figure out | An educated guess of the real value based on your sample data |
These differences stem from a fundamental concept in data: populations versus samples
What’ the Difference Between Population vs. Sample?
To really get parameters and statistics, understand the difference between a population and a sample:
- Population: This is the whole group you want to learn about. It could be all your current customers, all the potential customers in a certain industry, or everyone in a specific group of people (like young professionals). It’s the big picture, the whole universe of people you want to understand.
- Sample: This is just a part of the population. It’s a smaller group you carefully choose to represent the larger population. Think of it as a mini version of your target audience, a group you can actually manage to collect data from and learn about the bigger group.
A sample should accurately represent the population. This means thinking about things like age, gender, location, interests, and anything else that matters. If your sample is biased, your statistics won’t give you a good estimate of what’s happening in the whole population, and your marketing choices could miss the mark.
And once you’ve got your sample or population, you’ll need to make sense of the numbers.
Key Number Types: Mean, Median, and Standard Deviation
You’ll come across some important types of numbers that help you summarize and understand your data:
- Mean (Average): Add up all the values and then divide by the total number of values to find the mean. For example, if you’re tracking how many times per month people in your target audience visit your website, the mean would tell you the average number of visits for everyone in your sample.
- Median: Arrange all the values in order from smallest to largest to find the median, which is the middle value. The median is helpful when you have data that might be affected by extreme values. For example, if you’re looking at how much money customers spend, the median purchase amount might be better than the mean if a few big spenders are throwing off the average.
- Standard Deviation: This tells you how spread out the data is from the mean. A small standard deviation means the values are clustered close to the mean, while a large standard deviation means they are more spread out. For example, if you’re looking at how satisfied customers are, a small standard deviation means most customers feel about the same, while a large standard deviation means there’s a wider range of opinions.
How to Use Statistics to Estimate Parameters
Let’s see how these concepts work in practice.
Since it’s not always possible to measure something about an entire population, use statistics from a sample to estimate the parameters. This is where statistics really shine!
Your client, an online clothing store, wants to launch a new email campaign to promote their latest collection. Instead of sending it to everyone on their email list, test it on a smaller group of, say, 1,000 subscribers. Analyze the open rates, click-through rates, and how many people actually buy something from that smaller group to get a good idea of how the campaign might do when you send it to everyone on the list.
Different statistical methods exist to estimate parameters from statistics, but the main idea is to use the information from your sample to make a good guess about what’s happening in the larger population. This helps you make smart choices about your marketing without having to collect data from every single person in your target audience.
How to Tell if You’re Looking at Sample or Complete Data
As a marketer, you’re constantly bombarded with numbers. Revenue reports, social media metrics, customer feedback – all these contain either complete data (parameters) or sample data (statistics). Knowing which you’re looking at helps you make better decisions.
The Language Test
Pay attention to how the data is described. Absolute terms typically signal complete data, while less certain language suggests you’re looking at a sample.
Clear Signals in Your Reports:
Parameter | Statistic |
---|---|
“Total revenue: $1.2M” | “Average order value: ~$85” |
“All 12,543 subscribers” | “Based on 500 responses” |
“Every transaction in 2023” | “Estimated market share” |
Where Your Data Comes From
Your data source often reveals whether you’re looking at a sample or complete picture. Consider a standard marketing analytics setup:
Internal systems like your CRM or payment processor give you parameters:
- Exact number of customers
- Precise revenue figures
- Complete email subscriber count
Third-party tools usually work with statistics:
- Google Analytics samples large traffic volumes
- Social media reach estimates
- Market research percentages
The Certainty Check
Look at how confident the claims are in your reports. When Netflix says “63% of members watched Wednesday in its first 28 days,” that’s a parameter – they know exactly who watched. But when they say “viewers might enjoy similar shows,” that’s based on statistical analysis of viewing patterns.
This distinction becomes crucial when:
- Planning marketing budgets
- Setting campaign goals
- Reporting to stakeholders
- Making product decisions
Understanding what you’re looking at helps you know how much confidence to place in the numbers and what kinds of decisions you can reliably make with them.
The Pros and Cons of Statistics and Parameters in Marketing
Now that you can identify your data type, let’s examine when to use each. Let’s break down what works and what doesn’t with each approach.
Working With Sample Data
Think of statistics like a taste test. Instead of eating the whole pot of soup, you try a spoonful to know if it’s good. In marketing, this means testing your ideas with smaller groups before going all-in with your budget.
What’s Good About It
When Netflix wants to test a new feature, they don’t roll it out to all 230 million subscribers at once. They test it with a smaller group first. That’s statistics in action, and here’s why it works:
- It’s practical and won’t break your budget: Instead of surveying every coffee drinker in America about your new brew, you can learn a lot from talking to just a few hundred. Your insights come faster and cheaper.
- You can predict what will work: If 30% of your test group loves your new ad, chances are good it’ll perform similarly with everyone else. This helps you make smarter decisions about where to spend your marketing budget.
- You spot patterns more easily: You might notice that customers aged 25-34 are twice as likely to buy after seeing Instagram ads versus emails. These insights help you adjust your marketing to match what works.
The Downsides
But statistics aren’t perfect. Here’s where things can go wrong:
- Your sample might be skewed: Say you run a customer satisfaction survey and get glowing reviews. Great news? Maybe not – if only your happiest customers bothered to respond, you’re missing the full picture.
- There’s always some uncertainty: When your test email gets a 25% open rate, the actual rate with your full list might be anywhere from 20-30%. You need to plan for this variation.
- Reading the data wrong is easy: High website traffic sounds great, but if those visitors aren’t buying anything, you might be misinterpreting what the numbers really mean.
Working With Complete Data
Unlike sampling, parameters mean studying your entire audience. It’s like counting every single person at a stadium instead of estimating based on the sections you can see clearly.
What’s Good About It
Getting data from everyone sounds ideal. Here’s why:
- You get exact numbers: You’d know precisely how many customers you have and what they want – no guessing required.
- Your decisions are rock-solid: With complete data, you can move forward confidently, knowing exactly what works and what doesn’t.
The Downsides
But there’s a reason most marketers don’t chase complete data:
- It’s usually impossible: Try getting data from every single customer or potential customer who might want your product. Even Amazon can’t do that.
- It costs too much and takes too long: By the time you finish gathering all that data, the market has probably changed anyway. Plus, the cost would blow most marketing budgets.
Understanding these trade-offs leads us to proven strategies for using both types of data.
How to Use Parameters and Statistics in Your Marketing and Best Practices
Just understanding statistics and complete data isn’t enough – you need to know how to use them effectively. Here’s your practical guide to getting reliable insights without wasting time and money.
Smart Sampling Strategies
- Mix Your Sources: A footwear brand learned this the hard way – their Instagram data showed one story (young, urban customers), while sales data told another (35-50 year old professionals were the big spenders). Now they combine social, email, in-store, and sales data.
- Use Statistical Power: A retail chain’s 100-person survey seemed fine but couldn’t detect important differences between customer groups. Using power analysis, they found they needed 385 responses for reliable insights.
- Target Specific Segments: When analyzing different age groups or customer types, maintain enough samples in each segment. Target aims for 400 responses per segment to keep their confidence levels high.
Smart Measurement
- Focus on Leading Indicators: Track metrics that predict sales, not just describe them. A software company found pricing page engagement predicted purchases better than general page views.
- Use Comparative Metrics: Raw numbers can mislead. Look at ratios like:
- Engagement rate vs total followers
- Customer acquisition cost vs lifetime value
- Conversion rate vs traffic quality
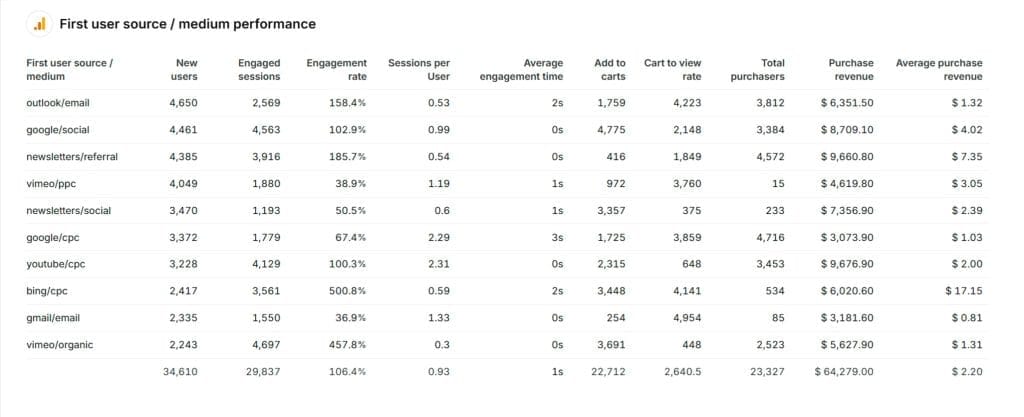
Quality Control
- Pilot Test Everything: Sephora avoided disaster by testing their survey questions with a small group first – terms like “skincare routine” meant wildly different things to different customers.
- Control for Time Bias: A restaurant chain discovered their satisfaction data skewed negative because happy customers were too busy to respond on weekends. They now weight responses by time and day.
Decision Making
- Set Clear Thresholds: Netflix uses specific viewing targets within 28 days to make show renewal decisions. Having preset triggers prevents analysis paralysis.
- Consider Full Costs: Amazon tracks not just conversion rates but total acquisition costs. Some “high-performing” channels weren’t worth their price when all costs were counted.
- Know When to Stop: Tesla launches with 80% confidence in their data rather than waiting for perfect information. They’d rather improve with real feedback than lose market advantage waiting for complete data.
This approach helps you balance the need for good data with the reality of marketing budgets and timelines.
Questions You Might Have About Statistics and Parameters
As you start applying these practices, you might have some questions.
1. How can I make sure the group I’m sampling accurately represents my target audience?
Use random sampling techniques to give everyone in the population an equal chance of being selected. You can also use stratified sampling to make sure your sample includes the important characteristics of your target audience.
2. What are some mistakes to avoid when working with parameters and statistics?
- Mixing up the two ideas: Always remember that parameters describe populations, while statistics describe samples.
- Thinking a small or biased sample represents everyone: Make sure your sample is big enough and represents your target audience well.
- Misunderstanding what statistical significance means: Just because something is statistically significant doesn’t always mean it’s important in the real world.
- Forgetting about the margin of error: When you make estimates or predictions, remember that there’s always some margin of error.
3. What tools can help me with statistical analysis?
Of course! Here are some popular ones:
- Microsoft Excel: Use this for basic data analysis and creating visuals.
- Google Analytics: Use this for website and marketing data.
- R or Python: Use these for more advanced statistical work.
- SPSS or SAS: Use these for specialized statistical analysis.
You can also find tons of online resources, courses, and tutorials to help you learn more about statistics and data analysis.
4. What is statistical significance, and why is it important in marketing?
Statistical significance helps you figure out if the results you see in your sample are probably true for the whole population or if they might just be due to random chance. It’s usually measured using p-values and confidence intervals.
For example, if you’re testing two versions of an ad and one has a higher click-through rate, statistical significance tells you how sure you can be that this version is actually better and not just doing better by chance.
Understand statistical significance to make good marketing decisions. This helps you avoid wrong conclusions from your data and makes sure your campaigns are based on real insights, not just random luck.
Next Steps
Start with these three actions this week:
- Audit Your Data Sources: Look at your last marketing report. Label each metric as sample or complete data. Are you making decisions based on the right numbers?
- Test Smart, Not Big: Before your next campaign launch, test with 5% of your audience. Use what you learn to improve your message before going all-in.
- Check Your Confidence: For your key metrics (conversion rate, engagement, sales), know your margin of error. If you’re not confident in the numbers, increase your sample size.
Better data leads to better decisions. The rest is up to you.
Make smarter decisions with the right marketing data today.
Start Your Free Trial Today